Among all the significant challenges chief data officers (CDOs) and data engineering managers face today, one of the biggest is dealing with centralized data teams. As the exclusive gatekeepers of data, these teams inadvertently create bottlenecks: their limited availability to fulfill organization-wide data requests hampers decision-making and data accessibility.
A shift toward new data management strategies is needed to overcome these challenges, and the data mesh architecture is one of the most promising solutions. This decentralized approach to data management favors domain-oriented data teams and self-serve data infrastructure for users to improve data accessibility and usability across the organization. It's particularly relevant to larger organizations that want to enhance their data operations without the constraints of centralized data management systems.
Yet, the decentralized nature of data mesh introduces its own challenges, particularly in ensuring data discoverability across dispersed data assets.
This article explores why you need a data mesh, its challenges, and the role a data catalog plays in overcoming them. It also examines the importance of integrating data catalog features such as data discovery, governance, documentation, and lineage tracking to amplify the efficiency and impact of your data mesh strategy.
Why Do You Need a Data Mesh Strategy?
A data mesh overcomes the limitations of centralized systems by ensuring data is more accessible, governable, and effectively used, which drives better business outcomes.
Challenges Created by Centralized Systems
A centralized approach to data management was initially considered the optimal solution for handling large amounts of data. However, it creates several challenges that negatively impact overall efficiency.
Centralized systems often struggle with maintaining high data quality due to the sheer volume of data they handle and the lack of domain-specific oversight. Without close management by those who understand the context and use of the data, errors, inconsistencies, and outdated information can proliferate, leading to poor decision-making.
When data is managed by a separate, centralized entity, users may not have visibility into how data is collected, processed, and maintained. This makes them skeptical about its accuracy and reliability, creating the issue of trustworthiness.
Data governance involves policies, procedures, and standards for data management. Counterintuitively, the scale of centralized systems and the diversity of data types and sources complicate effective governance, resulting in inconsistent data practices, privacy concerns, and compliance risks.
Centralized data management comes with bottlenecks and red tape. A single team handling all data requests leads to delays and restricts quick data access for decision-making, which hurts market adaptability. A uniform approach to data governance that doesn't cater to the unique requirements of every business unit further diminishes an organization's agility.
A centralized approach also creates issues with accessibility. With data siloed in central repositories, users across the organization may find it difficult to access the data they need when they need it. This can further slow down decision-making processes and hinder the organization's ability to respond to market changes and opportunities promptly.
How a Data Mesh Strategy Addresses Challenges of Centralization
The challenges of a centralized data strategy highlight the need for a shift toward a decentralized data management strategy like the data mesh. It addresses these problems by embracing four core principles.
- Domain-oriented decentralized data ownership and architecture advocates for decentralized data management within business domains to align data with domain expertise, enhancing accuracy and preventing silos. It encourages sharing data as a product and improving agility by allowing domains to quickly meet their needs, which leads to cost optimization and governance tailored to specific requirements.
- Data as a product involves managing data with strategic care to ensure it's discoverable, trustworthy, and self-describing. It democratizes data access, enhances discovery, and maintains data with a focus on value, which allows organizations to use data more flexibly.
- Federated computational governance merges centralized and decentralized approaches by setting global standards while allowing domain flexibility in implementation. It prevents governance from hindering agility by granting domain autonomy in a standard framework. It also reduces costs by eliminating redundant efforts and enhances data quality by customizing governance to domain-specific needs.
- Self-service data infrastructure for data consumption promotes a self-serve data infrastructure accessible to all organizational domains without needing specialized skills. It democratizes data access by enabling easy sharing and analysis, thus supporting quick decision-making and enhancing data discovery. Reducing reliance on IT and data specialists lowers costs and fosters flexible, innovative data use across the organization.
Roadblocks to Adopting a Data Mesh Strategy
However, all the benefits of a data mesh strategy come with their own set of challenges.
Unlike most solutions in the modern data industry, a data mesh is not a SaaS product that you can simply buy and implement in your organization. It's a strategy based on the principles explained above that can help you focus on data management from a decentralized perspective.
This means shifting toward a data mesh strategy requires a significant change in mindset in addition to restructuring organizational and operational models. Making this shift successfully requires overcoming resistance and cultivating a culture that supports data mesh principles.
For instance, shifting to a data mesh strategy requires adopting a product-oriented approach, which is challenging because teams must develop a consumer-centric mindset—designing data products that meet end users' needs, which is a new practice for many.
Furthermore, these products must be versatile and reusable across various use cases, demanding substantial efforts in standardization and governance. In terms of governance, ensuring compliance with both global and local regulations adds another layer of complexity as it involves navigating a maze of diverse and sometimes conflicting laws while keeping the data usable and accessible.
The challenges of designing data products are compounded by its decentralized nature.
When giving domains the autonomy to solve their own problems, organizations must ensure that they do not end up with multiple tools and technologies to deal with as this can impair interoperability and slow down the implementation of a data mesh strategy. Furthermore, organizations must also ensure they are effectively managing domain-oriented decentralized data and avoiding data silos, which would negate one of the biggest advantages of data mesh—easy data discovery and access.
The Role of a Data Catalog in Data Mesh Strategy
Although there is no silver bullet to solve all of these challenges, a data catalog can play an important role in mitigating them.
A data catalog can serve as a comprehensive inventory, listing every data product in an organization, irrespective of its source, to provide easy access to detailed metadata and descriptions for each data product. Within a data mesh strategy, a data catalog promotes data discoverability and facilitates the decentralized ownership and creation of data products as well as data consumption across diverse domains.
In other words, the data catalog is central to the data mesh principle of self-service data infrastructure for data consumption since it provides all the functionalities needed by both the producers of data products and their consumers. It facilitates domain-driven ownership, treating data as a valuable product, self-service capabilities, and federated computational governance. It weaves together the various strands of the data mesh framework, ensuring that data is not only discoverable, manageable, and accessible but also used in a manner that drives fast decision-making, efficiency, and competitive advantage. Without it, the aspirations of a data mesh strategy risk falling short of their promise.
That said, not all data catalogs are created equal. Some limit themselves to the basics explained above, while others offer additional features. According to Zhamak Dehghani, founder of the concept of a data mesh architecture, a data catalog should (ideally) have these features:
- Data governance management
- Data schema management
- Data versioning
- Data encryption, both during transit and at rest
- Automatic tracking of data lineage
- Metrics for assessing data quality
- Easy data discovery, cataloging, and publishing across stakeholders
- Advanced monitoring, alerting, and logging for data
Let's explore how a data catalog that ticks these boxes enables a data mesh strategy.
Data Ownership Management
In a data mesh architecture, each domain in an organization is responsible for its own data.
A data catalog enhances this principle by serving as a centralized repository where each domain can organize, describe, and manage its data assets according to its specific needs. Moreover, by centralizing metadata and providing a searchable inventory of data assets, data catalogs bridge the gap between scattered data points, thus enhancing data discoverability.
Data catalogs also establish a clear delimitation of data ownership. Each domain's data can be tagged and attributed directly to them, which facilitates domain-specific data management and governance while promoting accountability and transparency. Domains can define their data's metadata, usage policies, and quality standards, making it easier to govern and share their data responsibly.
For instance, Secoda lets you assign responsibility for a data product to an individual or team.
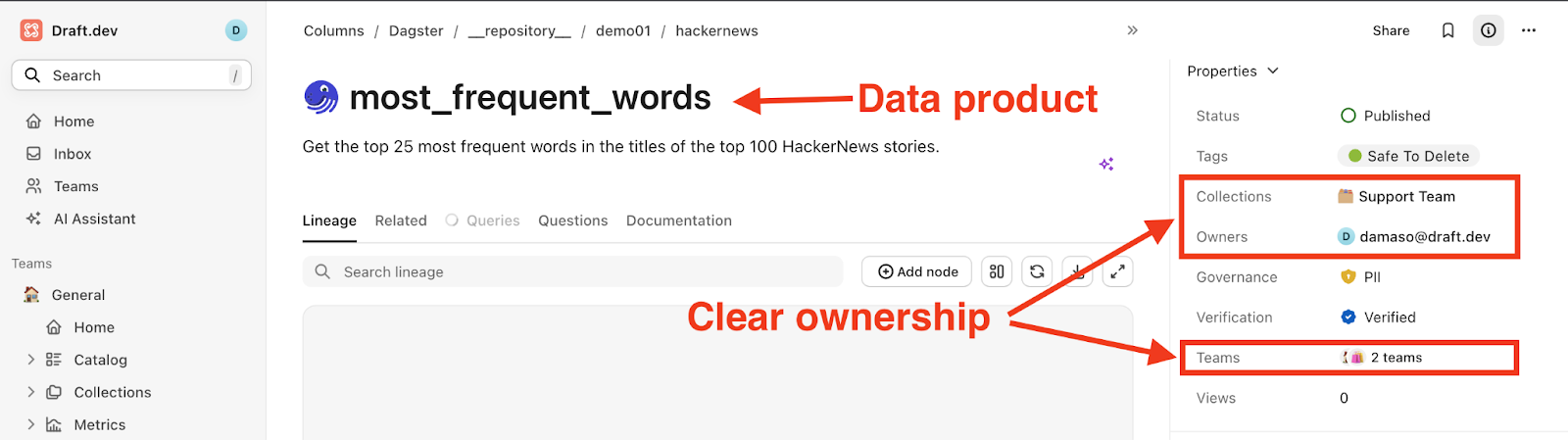
Improved Data Governance
A data catalog strengthens the federated governance principle by offering visibility into all data assets in the mesh, including their governance policies, ownership, and usage constraints.
It allows you to enforce compliance standards and governance practices at the domain level while ensuring these practices are consistent with the organization's overall data strategy. By providing a unified view of data assets and their governance context, the catalog facilitates effective governance across domains. This ensures that data is managed responsibly and in compliance with both internal policies and external regulations.
For instance, the screenshot below shows a simplified example of governance and usage policies in Secoda. The tag Safe To Delete
leaves no room for interpretation: this is a product that can be deleted, even though the governance field indicates that it contains sensitive data (PII).
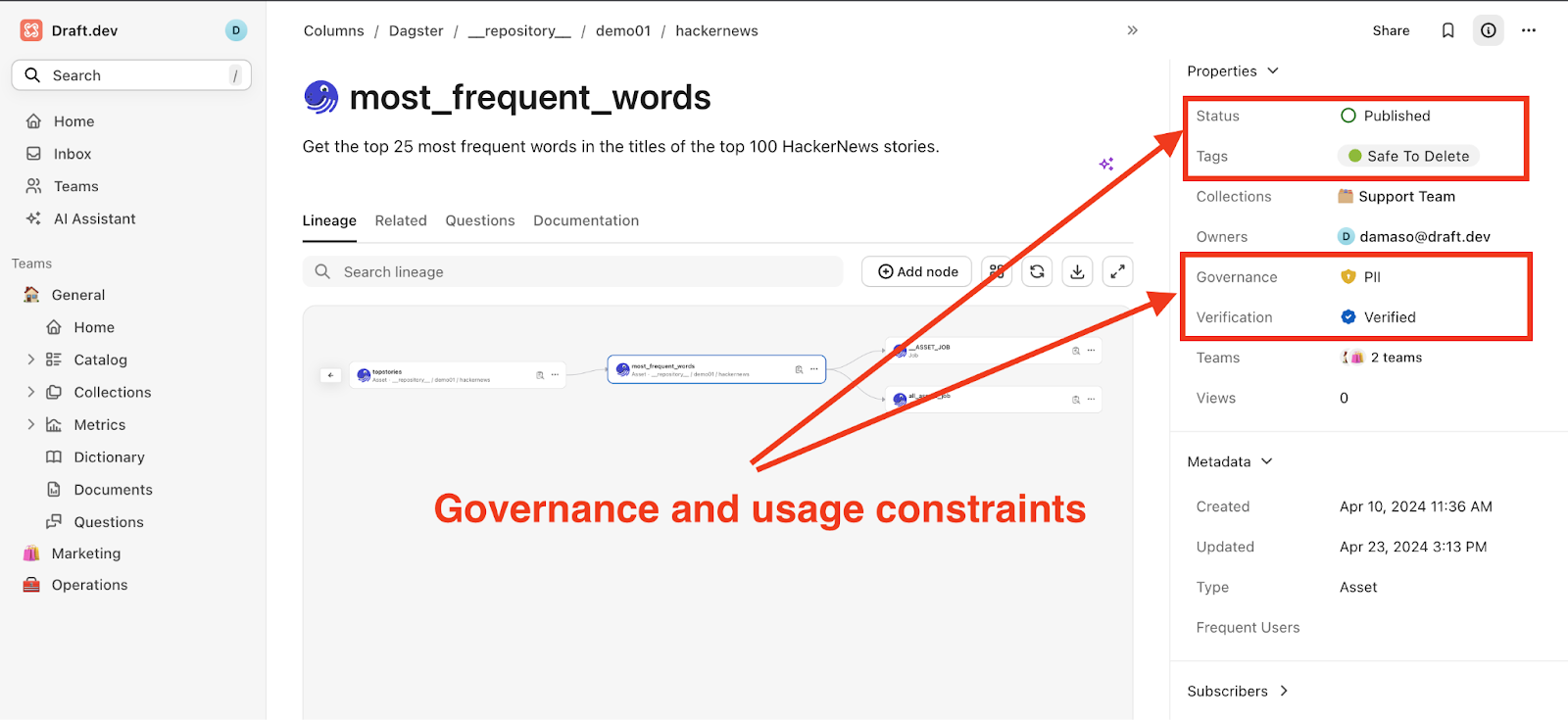
Capital One is a good example of how a data mesh framework is essential to distribute data ownership across business lines while maintaining a well-governed data practice.
Enhanced Data Discover
The self-serve data mesh principle aims to empower data consumers to access and use data without the constant intervention of data engineers or centralized data teams.
A data catalog helps realize this goal by providing a user-friendly interface where data assets are organized, searchable, and accessible. Users can independently explore the catalog, find the data they need, understand its context and quality, and access it according to predefined governance policies. This reduces bottlenecks and dependencies on specialized teams, fostering a culture of agility and autonomy in data usage across the organization.
This screenshot shows how easy it is to search for data products in a data catalog like Secoda:

Netflix is an excellent use case that shows the potential of the data mesh strategy to improve data accessibility and agility across an organization. Its data mesh allows the operational data from CockroachDB to be extracted once and then processed in the same pipeline in a variety of different ways.
Improving Data Trust
Treating data as a product means data should be accessible, usable, and trustworthy to its consumers.
A data catalog supports this by providing detailed documentation, descriptions, and lineage information, enhancing understandability and trust. This enables data consumers to easily discover and understand the data products available to them, akin to browsing a catalog for products that meet their needs. By enhancing the discoverability and usability of data, the catalog ensures that data products are designed with the end user in mind, improving their overall quality and relevance.
The screenshot below shows an example of data product documentation in Secoda. The consumer can gain an understanding of what the product is, where it comes from, and what it's used for to make an informed decision about whether this data product meets their specific requirements.
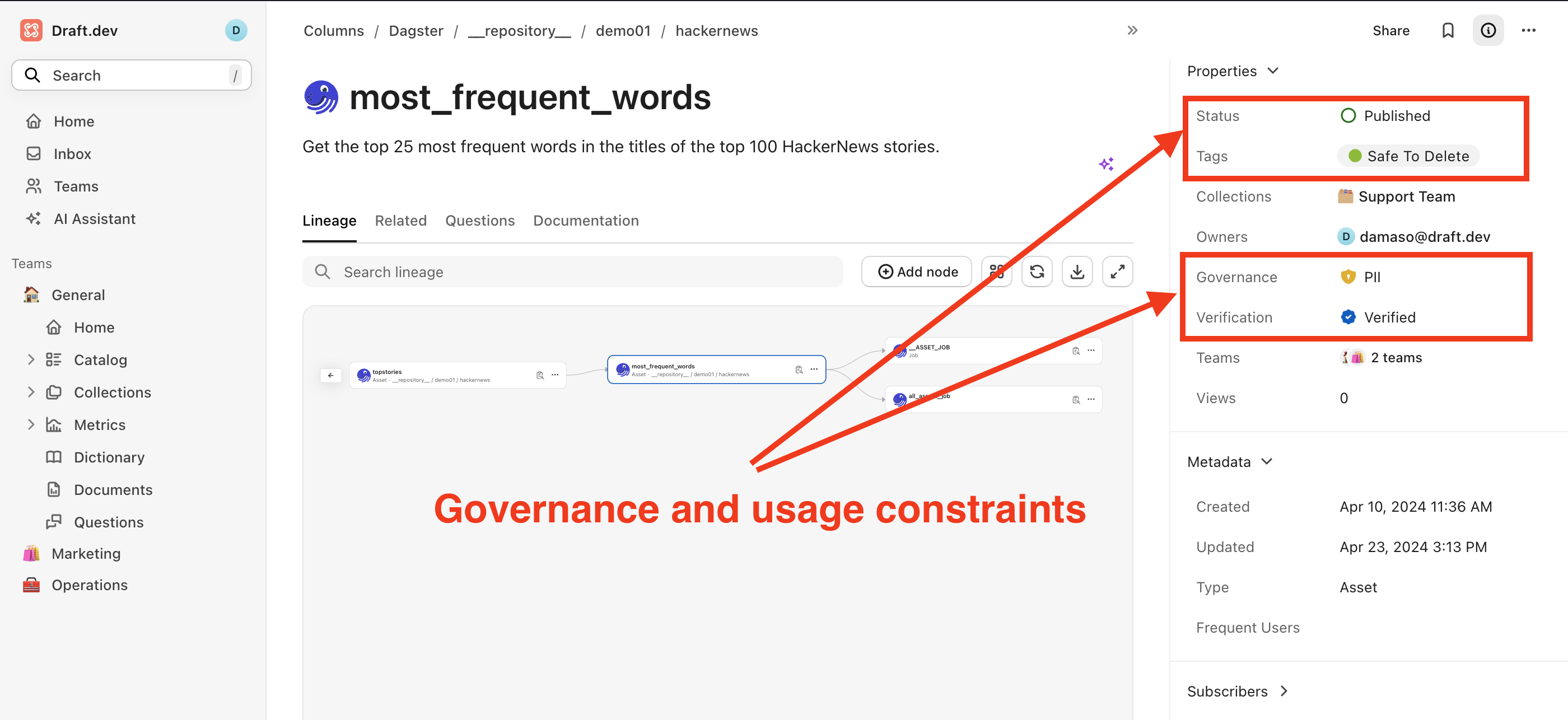
Final Thoughts
Forward-thinking data leadership is more than ready to let go of centralized data management. But while a decentralized data mesh strategy is appealing, it comes with considerable challenges too: adopting a product-oriented mindset and the change management it entails, ensuring governance, and effectively managing domain-oriented decentralized data.
A data catalog can help address many of these challenges. As you learned in this article, it's not merely a component of a data mesh strategy; it acts as the glue that binds data mesh principles together, enabling organizations to realize the full potential of their data products.
Secoda provides all the benefits of a data catalog and more. It consolidates all your data tools into a unified platform that provides a cohesive data mesh framework to enhance data discoverability, streamline workflows, and improve data governance. You can book a demo to learn more about how Secoda's integrated data catalog can unlock the full potential of your data mesh architecture.