10 Data Governance Best Practices for Banking and Finance
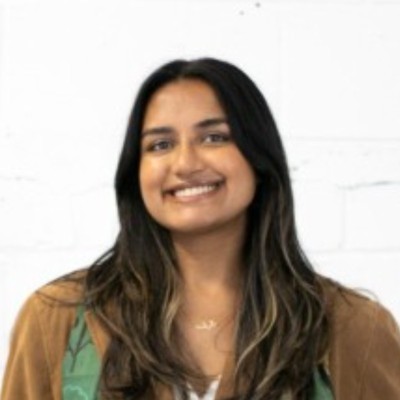
Data governance is essential in the banking and finance sectors due to the vast amounts of data generated at high velocity. This data often comes from multiple sources and departments, making consistency a challenge. For example, calculating average transaction durations across various branches can be difficult if each branch uses different methodologies. To effectively manage this complexity, organizations can benefit from understanding the importance of data governance and quality initiatives.
Without a robust data governance strategy, financial organizations cannot rely on their data for informed decision-making. Data governance is crucial for several reasons:
Implementing a successful data governance program can be complex due to various factors such as organizational culture, insufficient support from leadership, and resource constraints. Some common challenges include:
To ensure a successful data governance strategy, financial organizations should follow these best practices:
Develop a structured approach to managing data, outlining goals, principles, and components. This framework should include policies for data access, usage, and security to ensure everyone understands proper data handling. For more detailed guidance, consider exploring a data governance framework.
Set specific rules for data management, including collection, storage, processing, and sharing. For example, customer data should be encrypted and stored securely, accessible only to authorized personnel.
Assign roles such as data stewards, custodians, and owners to ensure accountability and proper management. For instance, a data steward may define quality standards, while a custodian manages storage and access permissions.
Outline processes to maintain high data quality standards, including:
Monitor key performance indicators to evaluate governance effectiveness and identify improvement areas. Metrics might include data accuracy rates, number of breaches, and compliance levels.
Technology plays a crucial role in supporting data governance activities. Investing in suitable tools and software can enhance efforts by automating workflows, managing permissions, and keeping resources up-to-date. For instance, data cataloging tools help manage metadata, while compliance automation tools handle regulatory requirements. Organizations can learn more about choosing the right data governance tool to aid their efforts.
Secoda, an AI-powered data governance platform, offers features such as:
By leveraging these technologies, organizations can overcome governance challenges and ensure data integrity and trust.
Secoda is a data management platform designed to improve data governance by offering a centralized system for discovering, cataloging, and managing data assets. It leverages AI to enhance data lineage tracking, access control, and automated documentation, ensuring data quality and compliance with regulations. This makes it an invaluable tool for data teams, analysts, and governance officers who need comprehensive control over their organization's data.
Secoda's AI-driven approach allows for automated data discovery and cataloging, providing a searchable data catalog that simplifies finding relevant data. It also offers enhanced data lineage by tracking the origin and transformation of data, which is crucial for governance and compliance. Additionally, Secoda improves data quality monitoring by identifying inconsistencies and alerting teams to address potential issues.
Secoda employs AI to streamline data management processes, making it easier for organizations to handle their data assets effectively. AI is used for metadata extraction, automatically enriching the data catalog with details like data type, format, and usage. This helps in creating a comprehensive view of the data landscape.
AI algorithms in Secoda also classify data based on sensitivity levels, aiding in data protection and compliance efforts. Furthermore, AI assists in data lineage mapping by analyzing data flows across systems, providing a visual representation of data movement. This capability is essential for understanding how data is used and ensuring compliance with data governance policies.
Secoda is beneficial for a wide range of users within an organization, each gaining unique advantages from its features. Data analysts and scientists can access and analyze data quickly by easily discovering relevant datasets within the catalog. This accelerates their ability to derive insights and make data-driven decisions.
Data governance teams benefit from Secoda's centralized monitoring and control, ensuring data quality and compliance with governance policies. The platform's features support effective data management practices, helping organizations adhere to regulations like GDPR and CCPA.
Business users can make informed decisions by readily finding and understanding the data they need. Secoda's intuitive interface and clear documentation empower users with varying technical expertise to utilize data effectively, enhancing overall data literacy within the organization.
By integrating Secoda into your data management strategy, you can streamline processes and improve outcomes across your organization. Discover how Secoda can transform your data management practices and enhance your organization's efficiency by exploring our comprehensive solutions.
Today, with the introduction of AI-generated visualizations and deeper integrations across the modern data stack, Secoda AI makes spontaneous data exploration and faster, more accurate answers a reality. Read Etai Mizrahi’s thoughts on how Secoda continues to eliminate barriers between curiosity and trusted insights.
Discover how Secoda’s new Monitoring and Catalog Application, now available as a Snowflake Native App on Snowflake Marketplace, helps data teams monitor data health, manage metadata, and improve governance directly within Snowflake.