AI Readiness: The Ultimate Guide
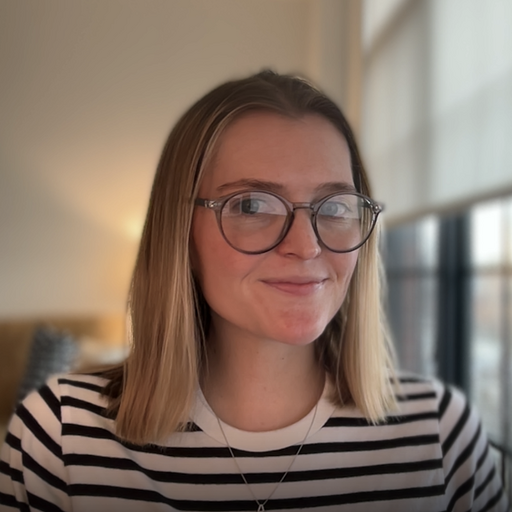
Let’s zoom out for a second.
For years, data governance was treated as a formality. It was manual, bureaucratic, and often siloed within legal or compliance teams. It felt like overhead. It slowed things down. And because it didn’t tie clearly to business outcomes, it was easy to push off.
But with the rise of AI and modern analytics, that mindset no longer works.
Today, if your data is incomplete, undocumented, or unreliable, everything else falls apart. Dashboards break. Models fail. AI systems hallucinate or perpetuate bias. And as executive pressure grows to deliver quick wins from AI, many data teams are left trying to build on shaky foundations.
Generative AI may have captured mindshare, but when it comes to budgets, data infrastructure still leads. According to IDC, organizations are prioritizing foundational systems over models because without that groundwork, nothing performs.
That’s where the AI Readiness Framework comes in.
To be ready for AI, your data needs more than basic collection. It needs governance. That means building on four foundational pillars: Reliable, Accurate, Usable, and Secure. These are the core areas to strengthen before generative AI can drive meaningful business outcomes.
AI depends on data you can trust. That requires systems and signals that ensure consistency across your pipelines:
You cannot achieve this level of reliability with manual updates or static documentation. It needs to be integrated into your tooling and maintained automatically.
The quality of the data you feed into AI models directly influences the results they produce. Accuracy starts with:
Tools like Secoda Monitors and dbt help teams not only catch issues but stop incorrect data before it reaches production. Real-time alerts make it possible to take action as soon as problems appear, especially when data shifts silently behind the scenes.
Even the most accurate dataset is useless if no one can interpret it. Usability is about giving people (and AI systems) the context they need:
Metadata should be treated as active infrastructure, not passive documentation. Start by enriching the data that supports your highest-value use cases. Broader standardization can follow once you’ve shown clear value.
AI adds new urgency to existing concerns around data privacy and compliance. Getting this right means embedding security directly into how your data is managed:
Security needs to be part of the system from the start. Anonymization, audit trails, and access controls should support velocity, not slow it down.
AI readiness starts with a clear-eyed look at your data foundation. Use this checklist to evaluate your team’s progress across the four pillars.
Is your data consistently trustworthy?
If reliability is weak, it becomes difficult to trust that the data feeding your AI models reflects reality.
Can you prove your data is correct?
Without built-in validation, errors can silently shape model behavior and go unnoticed until the damage is already done.
Can your team (and your AI systems) understand the data?
Even the cleanest data is unusable if people can’t understand it or find what they need.
Is your data properly protected and access-controlled?
Strong governance doesn’t just safeguard your data. It empowers your teams to move quickly without taking on unnecessary risk.
The more checks you can confidently mark, the closer you are to true AI readiness.
When preparing your data for AI adoption, it’s tempting to jump straight into model development. But without a strong foundation, even the best models won’t deliver results.
Here’s a phased approach to help you focus on the right work early on, starting with governance that actually supports AI.
You can’t govern what you can’t see. Start by bringing your documentation, tags, owners, and glossary terms into one unified catalog. This gives you visibility into what data exists and who’s responsible for it.
Focus first on high-impact datasets powering key models and dashboards. Assign ownership where it’s missing, and apply business-relevant tags. Use automation to avoid making metadata upkeep another manual task.
Instead of creating a new layer of governance roles, embed ownership within existing teams. This keeps things moving and makes adoption easier.
Choose tools that automatically capture lineage from ingestion through transformation to model training. Lineage should cover both table and column level dependencies, without requiring manual input.
This becomes especially important during model updates, retraining, or debugging. You need to know what changed, where it came from, and what it might affect.
Governance isn’t just about limiting access. It’s about enabling safe, secure collaboration. That starts with clearly defined roles, streamlined approvals, and sensitive data tagging.
Policies should be codified in your workflows, not added manually after the fact. For teams in regulated industries, consider anonymization and synthetic data approaches that preserve privacy while maintaining utility.
Build proactive data quality workflows. Start with lightweight validation rules on high-value datasets, then expand to cover drift and schema changes over time. Connect alerts to Slack or Teams so your team can act fast when issues arise.
Governance only works if people actually use it. Provide teams with automation, templates, and helpful defaults instead of review boards and approval queues. Build guardrails into the systems they already use.
Think of it as governance-as-a-service. It should guide people without getting in their way.
Once the groundwork is in place, you can begin layering in AI use cases with confidence. That might include semantic search across your catalog, using AI to generate documentation, or deploying lightweight models for internal tasks.
Start where value is clear and the risks are low. A strong foundation means each new use case is easier to support and scale.
Once the right systems are in place, the next challenge is scale. That’s where Secoda AI helps.
Secoda AI is like having a data analyst available 24/7—one who knows your data’s lineage, documentation, semantic layer, and quality status. Anyone can ask questions in plain language and get answers backed by context, not guesswork. It writes and executes SQL, pulls from your catalog and monitors, and explains how the data works.
For data teams, it means fewer repetitive requests, fewer one-off Slack messages, and less time spent tracking down owners, definitions, or upstream impacts. For business users, it means getting answers themselves faster and more accurately, without creating new bottlenecks.
With Secoda AI, you can:
Secoda AI makes governance feel less like a chore and more like a built-in productivity tool. It helps teams move faster, with better data, and prepares the entire organization for AI adoption on your terms.
Secoda’s Data Quality Score (DQS) brings the AI Readiness Framework to life. It breaks down your data assets across the four key pillars and gives you a single, actionable score.
You don’t have to guess where to start. Secoda shows you exactly what to improve, and helps you track progress as your data becomes more trustworthy, better documented, and AI-ready.
With automated suggestions and real-time scoring, DQS helps teams:
Trying to manage governance across multiple point solutions creates more problems than it solves. It leads to:
All of this slows teams down and creates friction that prevents AI from delivering results.
Secoda solves this by bringing everything into one platform, purpose-built for modern data teams that want to move quickly while staying compliant.
With Secoda, your team gets:
Instead of stitching together five tools and hoping they stay in sync, Secoda gives you one place to manage everything. It’s fast to implement, easy to maintain, and flexible enough to support any stage of your AI journey.
Governance is no longer just about compliance. It is the foundation for analytics, self-serve reporting, machine learning, and trusted AI.
While the AI market continues to grow, most organizations are still catching up. The biggest blockers are not talent gaps or model performance. They are upstream in the data.
Investing early in governance, metadata, quality, and infrastructure reduces risk, speeds up AI initiatives, and builds trust in outcomes. It also gives your data team the clarity and tools they need to deliver results on their own timeline, not just the executive’s.
If you’re ready to build a real foundation for AI, book a demo and see what Secoda can do.
Today, with the introduction of AI-generated visualizations and deeper integrations across the modern data stack, Secoda AI makes spontaneous data exploration and faster, more accurate answers a reality. Read Etai Mizrahi’s thoughts on how Secoda continues to eliminate barriers between curiosity and trusted insights.
Discover how Secoda’s new Monitoring and Catalog Application, now available as a Snowflake Native App on Snowflake Marketplace, helps data teams monitor data health, manage metadata, and improve governance directly within Snowflake.